How to "AI" - LLMs as a Commodity
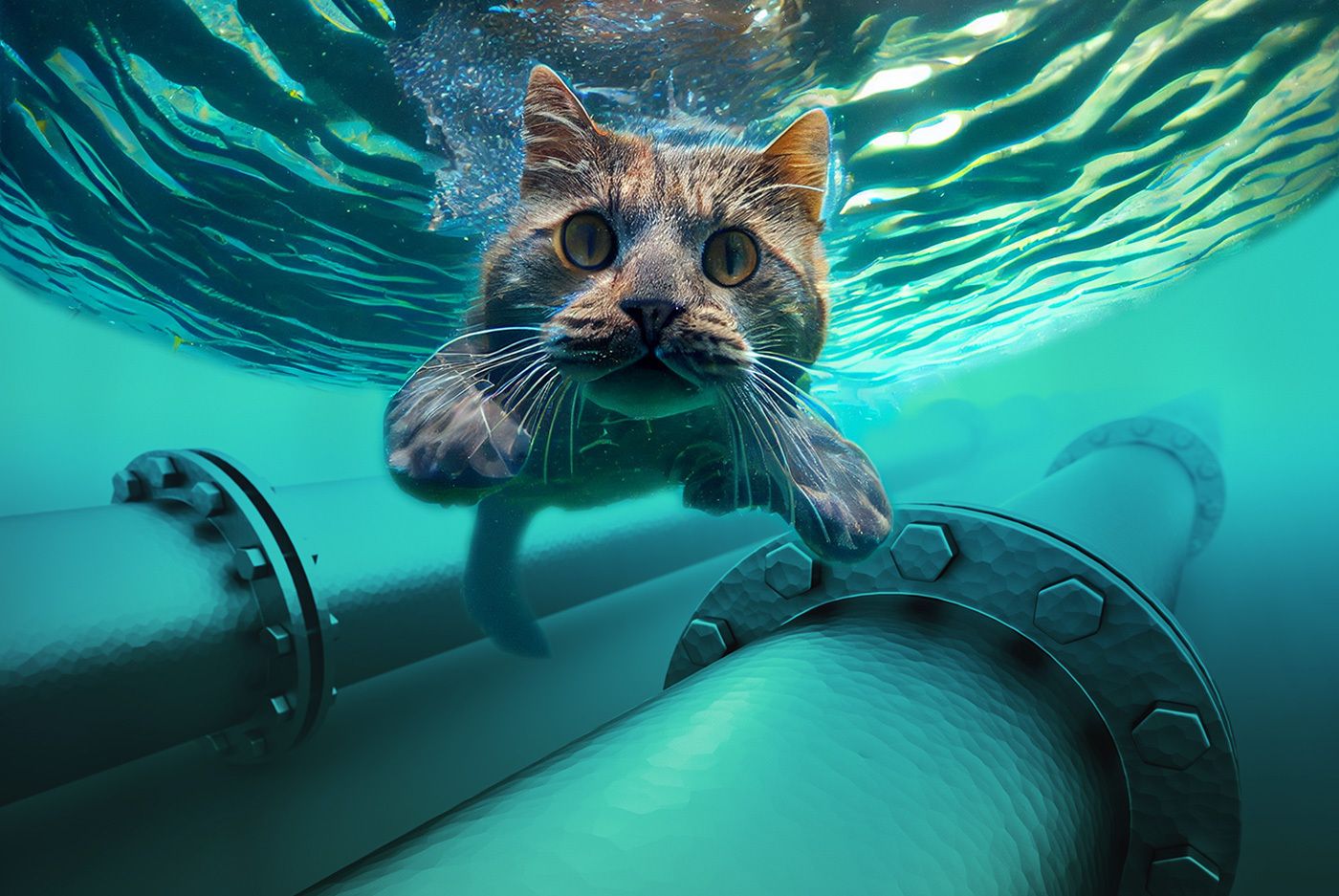
Some software is very expensive, some is cheap and some is free. And some types of software cost as much as several million in 2005 and can now be obtained for absolutely nothing.
Enterprise search has been on this journey. In the beginning, the sheer power of putting together a scalable system that you could stuff data into and, ideally, get it out of was worth hundreds of thousands of dollars to customers. And tens of thousands more in professional services to keep it all running (at least some of the time).
Solr, OpenSearch and Vespa are now (and have been for a long time) free in the true sense of the word and can do the same or more.
Is that why people don't make money from search anymore? On the contrary, but the focus has shifted drastically. Customization, scaling the system in heterogeneous environments or smart solutions for business problems are in the spotlight and are paid for. Core software development is the responsibility of the open source community.
The moral of the story: the actual software is (almost) never a revenue generator in the long run, unless it creates a quasi-monopoly for itself. Research, open source, disruptive competitors, all this will sooner or later cause such licensing models to collapse.
In the same way, even today, one should wisely refrain from building a business model on the premise of using LLMs (i.e. Large Language Models). An API wrapper around ChatGPT or a service that in reality only prompted Vicuna cannot last long. Even owning the most wonderful LLM in the world and making it available for money is probably not a sustainable business model in the long run. There is too much competition from different models, more and more of which are freely available or at least cheap to use.
Obviously, this does not bother the infrastructure providers like Microsoft and Amazon very much, they can earn a lot of money with overpriced GPU instances anyway.
But how can you create lasting and unique value in the generative AI space?
I argue that it works very similarly to search engines, document management systems, and other enterprise software: the added value comes from the knowledge and data that users provide to the software and the way you handle it as a service provider.
My top 4 ingredients for unique solutions that will still be worth their money in 5 years:
- Technical understanding
Yes, you have to know what you are doing, how to use, adapt, roll out the appropriate technologies. Even this is lacking in regards to LLMs especially among those who are the loudest on LinkedIn. - Understanding the problem
What is the problem we are solving? What business process is being improved? How does the customer save money or make money? Don't look for the problem to the technology, look for the technology to the problem is the name of the game here. - The right data in the right form
"Data is the new oil." This is true insofar as data is the fuel and lubricant of such technologies. What data can be used to fine-tune an LLM so that it becomes an expert in European regulations? What are the best text sections for sample prompts? All these treasures exist at the customer's site and need to be put into the right form for the LLM technology to benefit from them. - User interactions
A big opportunity of machine learning algorithms in general is that you can build feedback loops to the user. The user doesn't like an answer? His "thumbs down" can directly become a negative example for the next fine-tuning. A user edits the output of the LLM a bit? Great, now we have a positive example or an extension for the prompt.
In "service provider circles," people like to talk a lot about "innovating with the customer." This integration of technical expertise with data assets and process understanding on the part of the customer is how we think of this at NEOMO.