ChatGPT and the oil spill
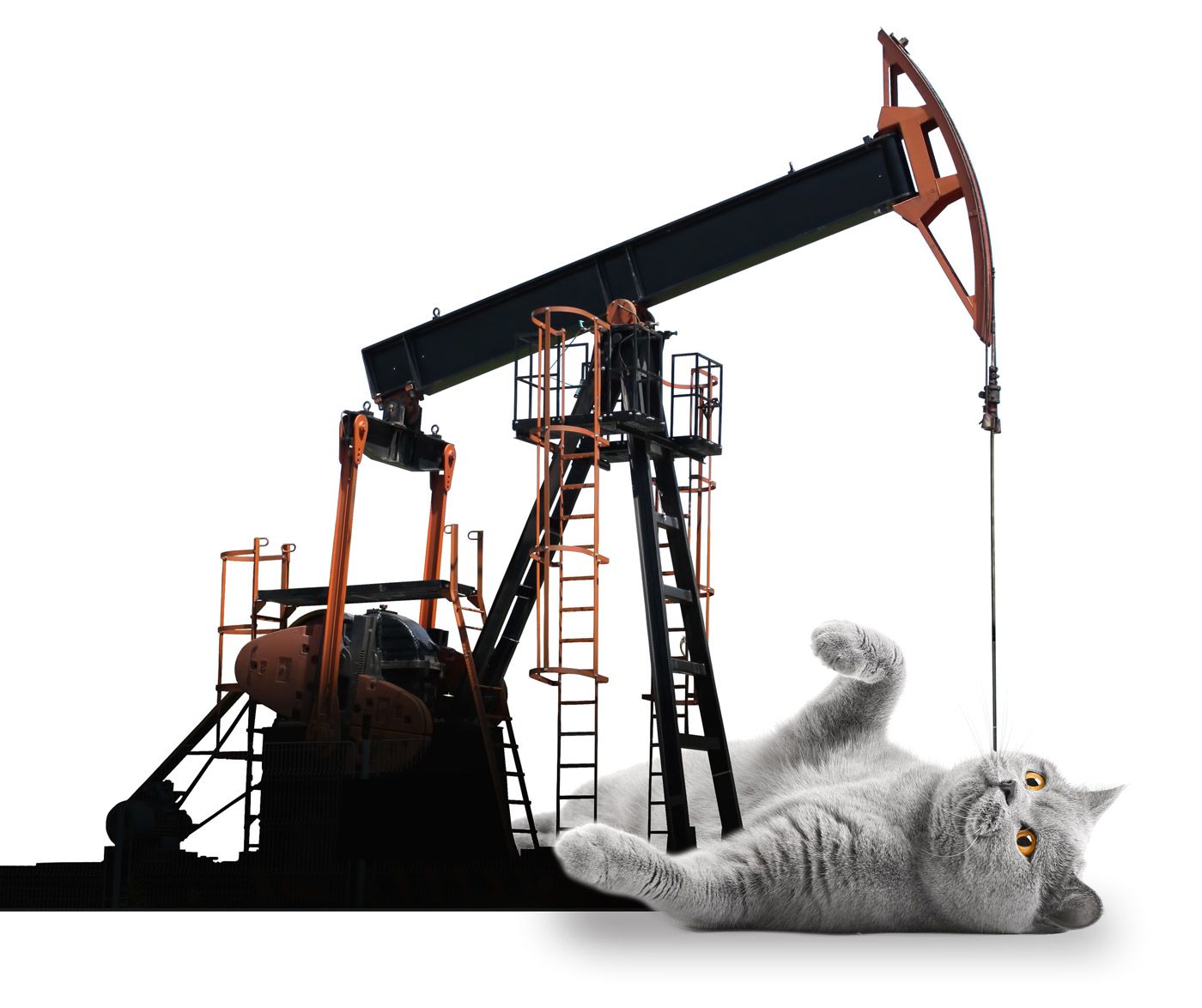
"Data is the new oil," said British mathematician Clive Humby in 2006. He probably didn't realize how right he was and how far this analogy can be taken.
- Data is like oil, without processing it is useless.
- Data is like oil, essential processes are driven by it.
- Data is like oil, it is - in a sense - bad for the environment.
- Data is like oil, it is valuable, though available in relatively large quantities.
But one of the key differences is that, unlike oil, data doesn't deplete. You can use the same data sets over and over again in different contexts.
Classical machine learning has always been about collecting and, more importantly, preparing data first. Automatic translation, for example, hinges on the existence of so-called "alined texts", i.e. documents that are available in two different languages and can ideally be compared sentence by sentence. On this basis, a machine learning model can then be trained to spit out the (often) correct counterpart also for new sentences. In the contest of such systems, the decisive factor was often who had the cleaner and larger data sets and not who had the better algorithm or the better technology.
The technical approaches are often based on freely available research anyway; it's the data that makes the difference.
With deep learning, this hunger for data has become much bigger. While previous methods worked quite well with 100 positive examples per category (e.g. 100 financial news vs 100 political news to train a classifier), deep learning methods needed at least one order of magnitude more. But then suddenly something crazy like image search or image categorization started to work.
With Large Language Models (and other forms of generative AI) we have ignited the next level of data craving. All the talk here is really about billions and then some. Granted, this input data is just uncategorized "tokens" instead of manually presorted documents, but the amounts of data used are still gigantic.
A key difference, however, is that in the case of ChatGPT and other such models, someone else has collected all sorts of multi-colored data for me and mixed it into a mash - an oil spill, so to speak.
As a result, the model can answer almost any question without any further data input, but conversely cannot guarantee truly reliable, accurate, or even reproducible answers for any question. The big data mush spits out an answer to almost everything, but the thinner the data was in the training set, the more questionable the answer becomes.
Huh, but ChatGPT was trained on all the knowledge in the world, wasn't it?
Even if it were (both "total" and "knowledge" and "world" are probably not appropriate terms), it needs not just one document on each topic, but a great many to end up with factually correct answers. You can see this very nicely if you go a bit to the "periphery" of the world knowledge: If there were few documents on a topic in the training set and if one perhaps even surprises the system with aberrant query languages such as German, then hallucinations are never far away:
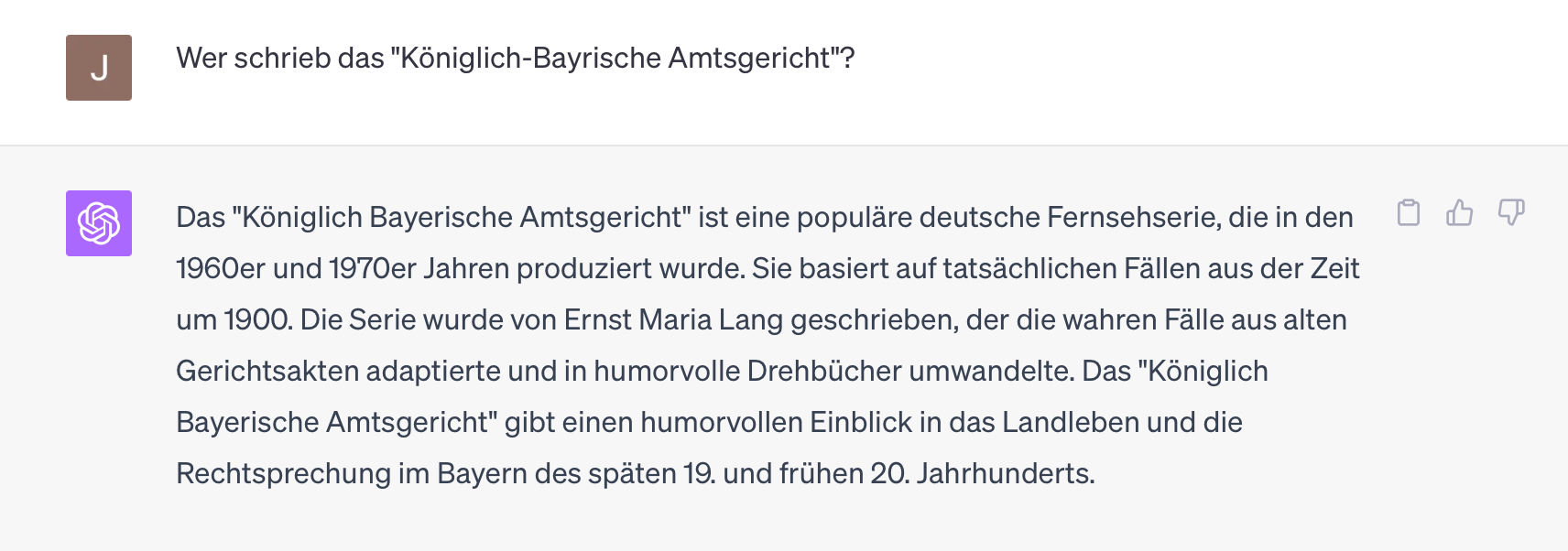
No, that is not correct, Georg Lohmeier wrote this series and the cases are all set in 1911/12.
But maybe the hyphen was confusing, in the original it is written without it:
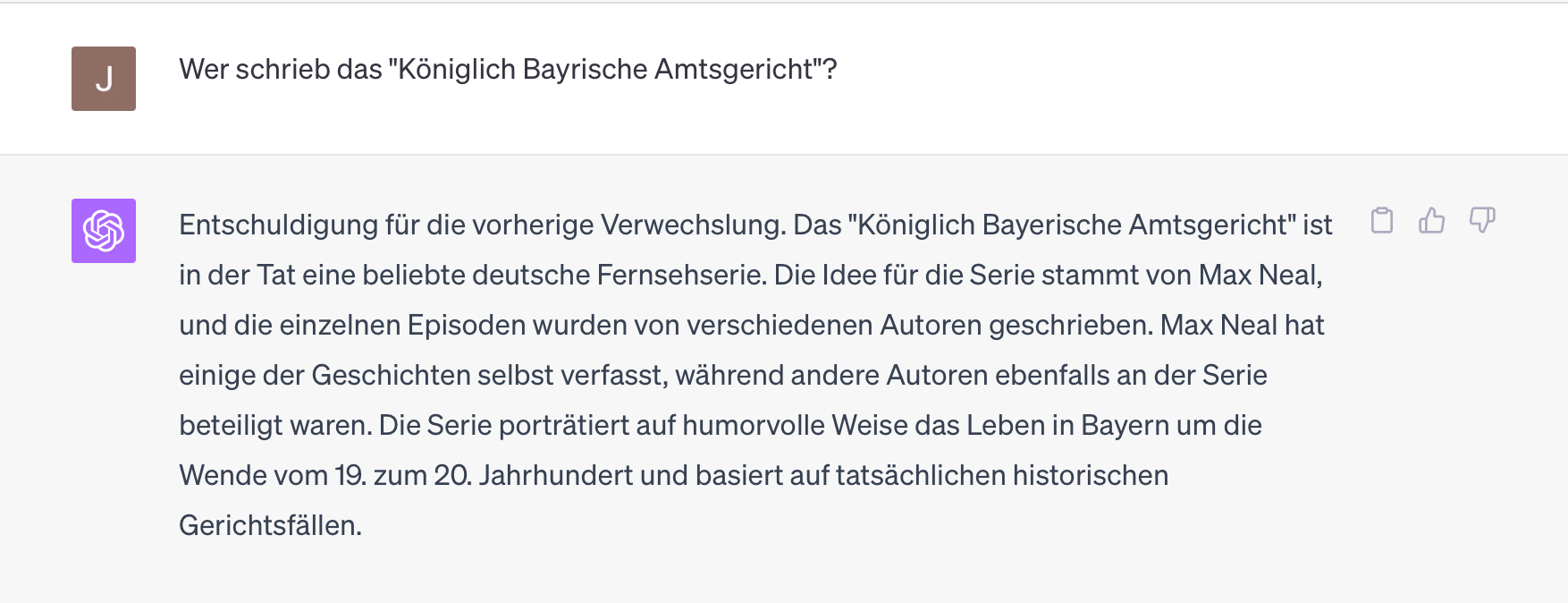
No, that is equally wrong. Interestingly, the model remains in the correct "environment", both Ernst Maria Lang and Max Neal are humorists from Bavaria in the broadest sense. But can the hyphen cause such a reinterpretation?
Let's rather try this again with a literal repetition (in the same chat window):
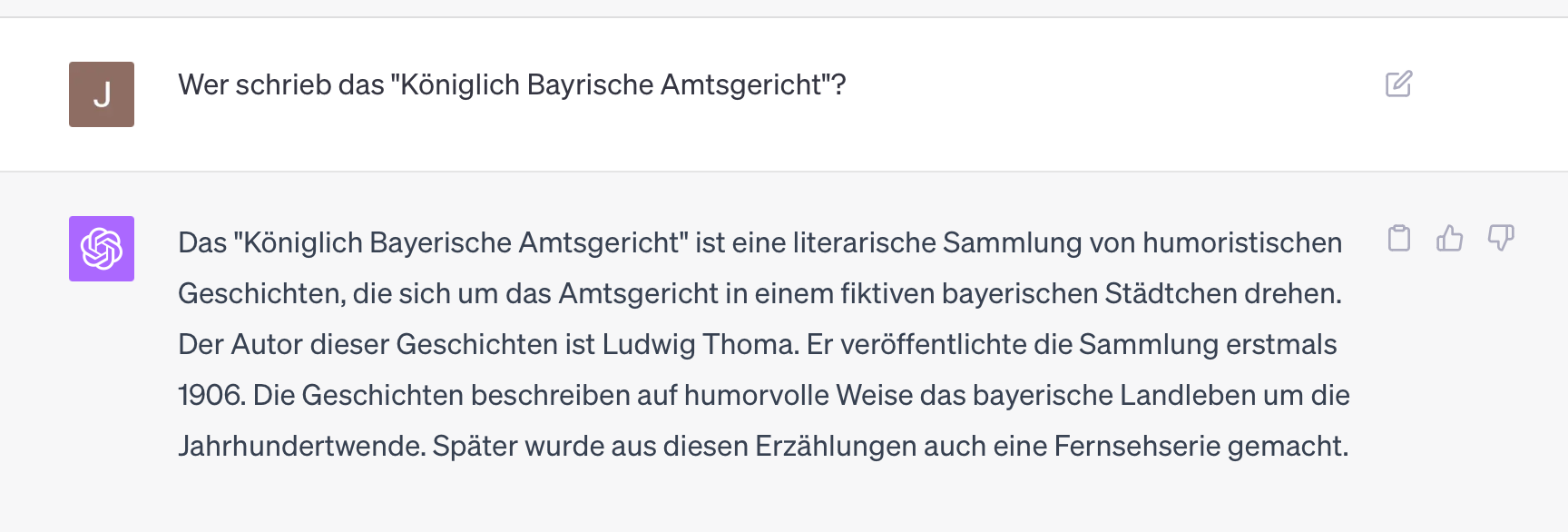
Ah, another Bavarian humorist, again the wrong one. For all mentioned gentlemen (including the real author) Wikipedia pages exist and also other information in the net. Nevertheless, ChatGPT-4 (in the very latest manifestation from Sep 12, 2023) happily fantasizes in different directions.
If we move a little bit more into the center of the world of ChatGPT, the answers become correct, consistent and above all repeatable again:
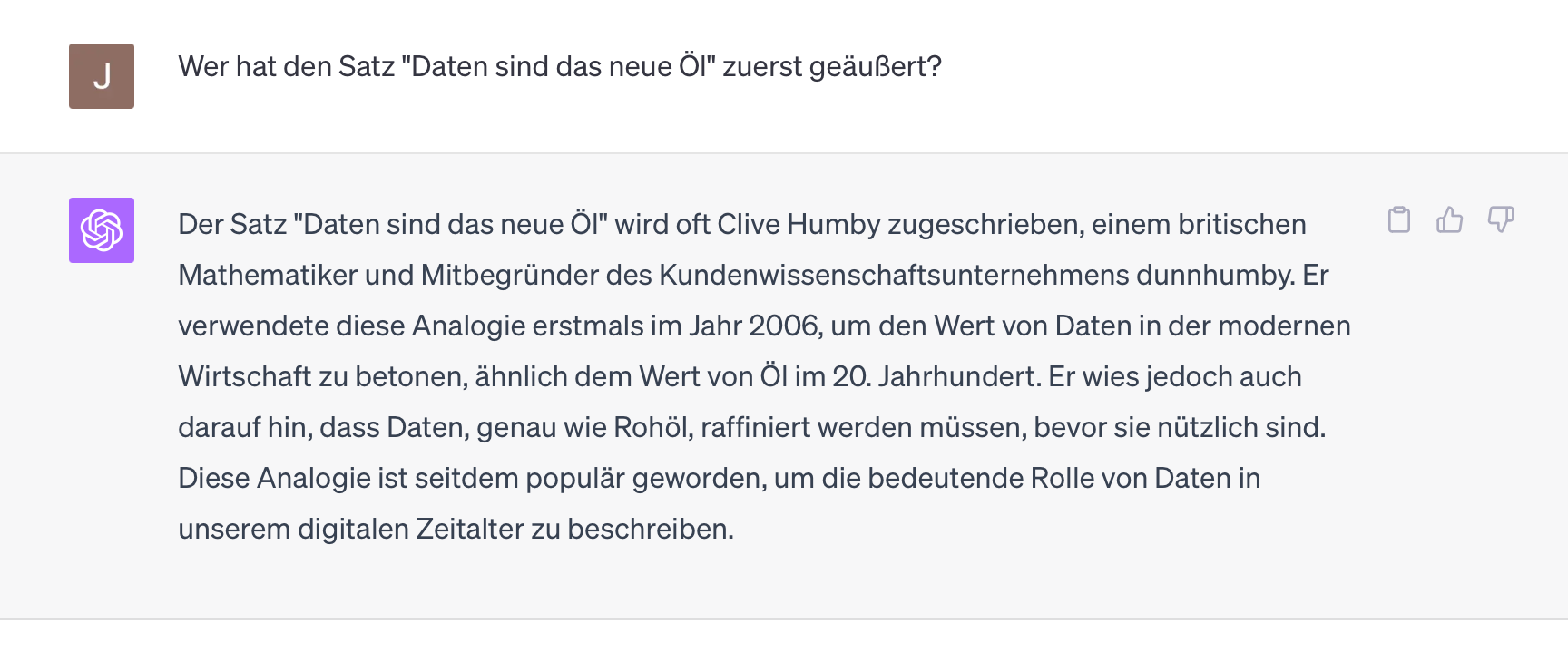
Every repetition of this question produces (for me, as of today) the same answer. And even the English version is consistent and virtually a 1:1 translation of the German one:
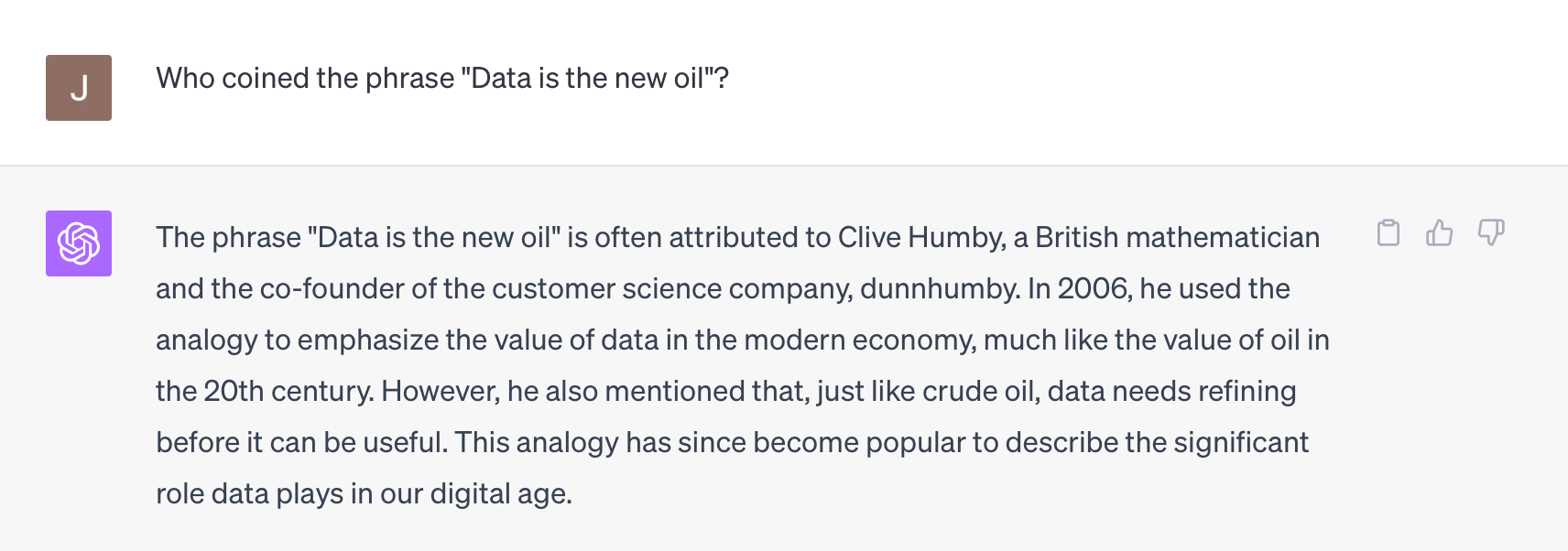
But does it matter? Is anyone really interested in the "Königlich Bayerisches Amtsgericht"?
Probably yes, but the more substantial problem is that countless start-ups are founded in extremely narrow niches with the claim to build a great "AI-powered" product for that niche, which in reality often means that these products send prompts to ChatGPT in a more or less imaginative way and then process its output.
The aforementioned example documents vividly what quality can be expected from such approaches once you leave the lush core of OpenAi training data.